Introduction
UX research is essential to understanding user behavior and improving the user experience of products in the fast-paced digital world. The role of artificial intelligence (AI) in accelerating insights is evolving along with the field of UX research. Researchers can now delve deeper into user behavior, preferences, and motivations, enabling them to understand better and improve the user experience with AI-powered tools and technologies. That is why I will explore 5 best applications of AI in UX research to accelerate insights in this article.
First, always ask AI system to cite primary sources and then go check those sources! Secondly, use tools specifically designed for information seeking such as Perplexity or ScholarAI. Thirdly, when asking AI tools to complete research related documentation provide them with a template as a starting point. Fourthly, AI-powered predictive analytics can forecast user behavior and provide recommendations for enhancing the user experience. And finally, when asking AI tools to create charts ask the tools to follow data visualization best practices. By harnessing the power of AI in UX research, companies can gain a competitive edge by understanding their users better and creating more engaging and personalized experiences.
Benefits of Using AI in UX Research
The integration of artificial intelligence (AI) into UX research offers numerous benefits that significantly enhance the way researchers understand user behavior. The ability to process vast amounts of data at unprecedented speeds is one of the main advantage. It might take a lot of time and resources to gather and analyze user data using traditional approaches. AI systems can quickly analyze user interactions, preferences, and feedback across multiple platforms, providing researchers with insights that would otherwise take weeks or months to uncover. It both saves time and allows for real time adjustments to products based on user feedback.
Another compelling benefit of using AI in UX research is the potential for uncovering hidden patterns and trends that might not be immediately apparent to human researchers. AI algorithms are designed to find correlations and anomalies in a large datasets, so that the team can make actionable insights that informs their design decisions. For instance, For example, machine learning models can analyze user behaviour as time goes on and identify when preferences change, or when new trends start to emerge. With this level of insight companies are able to proactively adapt their strategies, ensuring that their products meeting user needs and expectations.
Moreover, AI enhances the scalability of UX research efforts. While businesses grow and expand their product offerings, the volume of user data also increases. AI tools can handle this amount of influx of information perfectly, allowing teams to generate high level of insights without requiring proportional increases in manpower. With AI, UX researchers can put more of their efforts on strategic decision-making and creative problem-solving, rather than getting stuck in the data collection and analysis. This change not only makes process more efficient, but it also encourages more innovation in the UX research process.
Key AI Techniques Used in UX Research
Multiple AI techniques are revolutionizing the landscape of UX research, each making a distinct contribution to our knowledge of user behavior. One of the most popular techniques is machine learning, enable systems to learn from data and get better at their predictions over time. In UX research, machine learning algorithms can analyze user interactions to identify patterns that inform design improvements. For example, clustering algorithms can segment users based on behavior, allowing researchers to personalize experiences for different user groups effectively.
Natural language processing (NLP) is another critical AI technique that plays a significant role in UX research. NLP allows machines to interpret and understand the human language which is very useful for analyzing qualitative data such as surveys, user feedback, and reviews. Sentiment analysis allows researchers to explore user emotions and opinions regarding a product, in order to gain more deep insights on user satisfaction and areas for improvement. This technique helps bridge the gap between quantitative data and qualitative insights, providing a complete view of user experience.
Another area that Computer vision really excels in is UX research, especially in analyzing visual content and user interactions with graphical interfaces. Through image recognition and analysis, AI can assess how users interact with visual elements of a design, from buttons to images and layouts. It will help designers to reveal which elements are getting most attention, and which may be overlooked, ultimately guiding design decisions to improve usability. With the use of these AI techniques, UX researchers can better understand multifaced user behavior and make more informed design strategies.
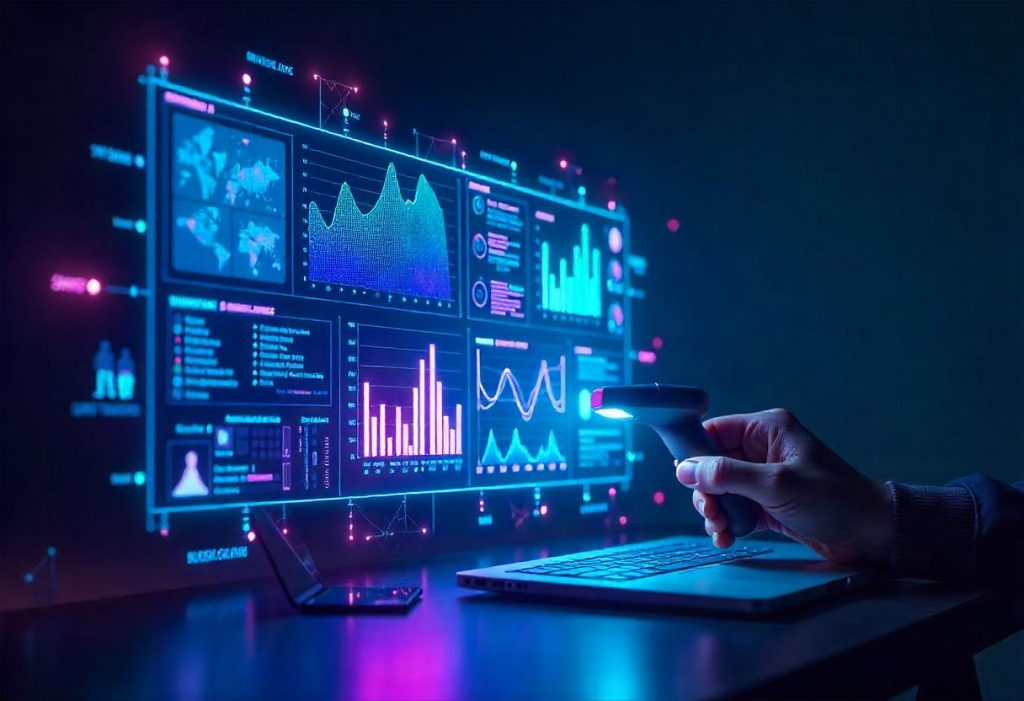
AI Powered User Behavior Analysis
Researchers’ observations and interpretations of user interactions are being revolutionized by AI-powered user behavior analysis. Researchers can examine user data and forecast future trends and behaviors by utilizing machine learning techniques. For example, predictive analytics can help identify potential bounce points in user journeys, allowing designers to proactively address issues that may hinder user engagement. This proactive strategy raises overall satisfaction and retention rates while also improving the user experience.
AI can also make it easier to analyze behavioral data on a large scale. Traditional methods often rely on small sample sizes that may not accurately represent the broader user base. AI tools, on the other hand, are able to analyze data from thousands or even millions of users, giving a more complete picture of how user behave. This feature enables researchers to identify key trends and outliers, so design decisions will be made backed up by robust evidence, rather than casual observations. This means that companies can then create more effective and user-centered designs.
Furthermore, AI supports continuous learning and adaptation in UX research. As new data is collected, AI models can be updated to reflect changing user behaviours and preferences. Through this iterative process user experiences stay relevant and aligned with changing user expectations. Continuous analysis of user behavior keeps businesses on the curve and able to offer things as per dynamic demands of the user, ultimately driving higher levels of user engagement and satisfaction.
Learn More Secrets: Integration of AI-Powered Financial Robo-Advisors: Enhancing UX in Fintech App
AI-Driven Sentiment Analysis for UX Research
AI-powered sentiment analysis is revolutionizing UX research by providing researchers with a powerful tool for understanding user emotions and attitudes toward products and services. Sentiment analysis uses natural language processing techniques to process vast amounts of textual data, such as user reviews, social media comments, survey responses to find out the general ‘sentiment’ expressed by users. This analysis can be used to learn how users perceive certain features or features of a product, which will help designers to understand where to improve.
The huge benefit here for UX researchers is being able to proactively understand what users think of a product (or website) in real time. Companies can quickly identify emerging issues or trends regarding user experience through monitoring social media and other online platforms. For instance, sentiment analysis can help in determining the degree of user dissatisfaction and highlighting certain issues brought up by them if a new feature receives overwhelmingly negative feedback. This immediate feedback loop lets teams know what they need to improve in real time, giving teams the ability to make key decisions and implement changes quickly, which makes for a really great overall end user experience.
Additionally, sentiment analysis gives better insights than ratings or scores. Researchers can categorize sentiments as positive, negative and neutral to find more specific user pain points and to be able to uncover nuanced opinions. This level of details provides a clearer understanding of user needs and expectations, enabling designers to design solutions that address these issues effectively. In the end, AI driven sentiment analysis turns UX researchers into great product designers as they learn how to create products that users will love emotionally and to increase loyalty and satisfaction.
Personalization through AI in UX Research
Personalization is one of the most crucial part of modern user experience, and AI is the backbone enabling tailored experiences for users. By analyzing user data, AI can identify individual preferences and behaviors, allowing companies to create customized experiences that resonate with each user. For example, e-commerce platforms, can use AI algorithms to make product recommendations based on a user’s browsing and purchase history. This level of personalization increases engagement and conversion rates in order to improve user satisfaction.
Real time adaptive interfaces derived from the user behavior can also be facilitated by AI. For example a website which changes layout, content, or the navigation according to how a user interacts with it. Such a flexible UX gives users the most relevant information and features to meet their needs, making it more usable and satisfying. With continuous learning from user interactions, AI systems can improve their personalization strategies, leading to increasingly tailored experiences over time.
In addition, the AI personalization extends beyond individual users to encompass broader audience segments. Using clustering methods, the researchers can group users with similar behavior and preferences together, allowing for more targeted marketing and design strategies. Such segmentation helps businesses to provide relevant experiences tailored to the individual needs of each user profile, leading up to increased engagement and loyalty. However, UX research processes could become increasingly personalized in nature following rapid developments in AI, opening up endless possibilities for creating compelling design based on user-centered design.
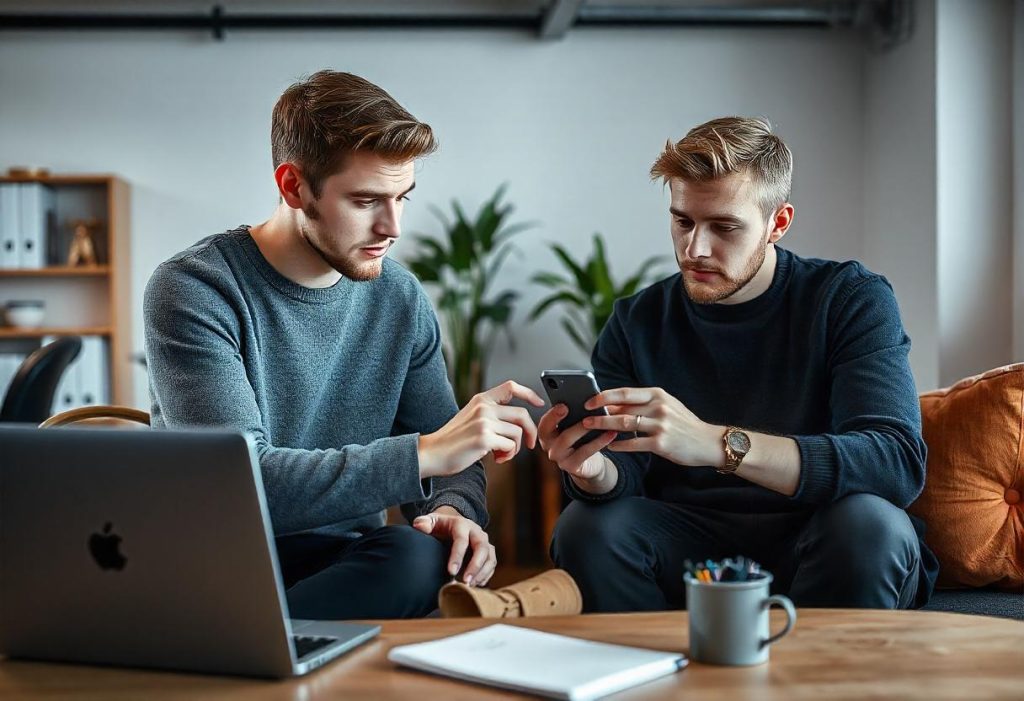
AI-Based User Testing And Feedback
AI-based user testing and feedback mechanisms are revolutionizing the way researchers gather insights about user experiences. Usability testing has traditionally used small focus groups, and manual observation that can miss the full depth of feedback potential. But AI tools are able to automate and scale this testing process, allowing researchers to collect data from more diversified user group. This scalability ensures that the insights gathered are more representative of the overall user base, resulting in better design decisions.
Furthermore, AI can make the user testing process highly efficient by analyzing user interactions and giving valuable insights in real-time. By utilizing tracking tools and heatmaps, AI can record ways that users interact with a product- identifying common pathways, click patterns, pain points etc. This data-driven approach gives provides researchers clear guidance on usability issues, where they can identify areas that need to be improved. And leveraging AI for user testing, companies will be able to simplify their product design process and creating even more intuitive and user-friendly products. In addition, AI-driven feedback mechanisms can improve ongoing user engagement and input.
For instance, some chatbots on the basis of AI are able to take user feedback through the conversational interface and provide a more interactive and engaging experience. Real time feedback from users on their experiences enabling researchers to collect insights continuously. This continuous feedback cycle allows the product to continuously update based on users’ expectations, which enables a culture of user centric design and innovation.
Case Studies: Showcasing Successful Implementation of AI in UX Research
Several companies have successfully used AI in UX research in their processes and this has improved users experience and product design significantly. One notable example is Airbnb, which utilizes machine learning algorithms to analyze user interactions on its platform. By discovering booking patterns and user preferences, Airbnb can provide personalized recommendations to users, ultimately enhancing their overall experience. This data-driven approach has not only improved user satisfaction but has also helped the company increase bookings and revenue.
An equally interesting case study is the one with Spotify that uses AI to generate personalized playlists for its users. By analyzing listening habits and preferences, Spotify’s algorithms can create tailored playlists that resonate with individual users. Such personalization is a key driver of user engagement, because users are more likely to explore and enjoy music that they like. Spotify’s successful AI driven personalization strategy shows that AI can completely change the user experiences in the music industry.
Amazon is leading the way in use of AI for UX research in e-commerce. Amazon uses algorithms that analyze user behavior and preferences to recommend products that align with individual user’s interests. This strategy not only improve the shopping experience as well as it significantly boosts sales. Amazon has set a standard for use of AI in UX research by refining its AI systems based on user feedback and behavior iteratively.
Challenges and Limitations of AI in UX Research
Despite the numerous advantages of using AI in UX research, there are also challenges and limitations that researchers must navigate. One of the largest problems with AI algorithms is the possibility of bias. Insights produced from AI models trained with data that doesn’t reflect the diverse user population used can be skew. This biasness can lead to designs that don’t fulfill the needs of all users and as a consequence, the product is less effective. So researchers must be careful to make sure their data sources are inclusive and represent the broader user base.
AI lacks UX research intuition, which complicates interpreting AI-generated insights which is an another limitation of AI in UX research. AI can analyze huge amounts of data and identify patterns, but they rarely have the ability to convert research findings into actionable design strategies. In order to take advantage of AI insights, researchers need to have strong understanding on both the technology and the user experience principles. Additionally, the reliance on AI tools may lead to an overemphasis on quantitative data, potentially overshadowing qualitative insights that are equally important in understanding user needs.
Last but not least, there are ethical aspects surrounding the use of AI in UX research. To keep user trust, we need to do the collection and analysis of user data transparently and responsibly. It has become a new reality that users are increasingly concerned about privacy and data security. Businesses must prioritize ethical practices, collect AI driven insights obtained with consent and understanding of users, and develop the respectful and trustworthy relationship with the audience.
Conclusion
From improved data analysis to better personalization, AI is changing how researchers are approaching user experience. Using AI, companies can understand user behavior more deeply and build products that truly reflect their users. The future prospects of AI in UX research are promising, with advancements in technology paving the way for even more innovative approaches to understanding users.
As I look ahead, I can only expect AI continue to have significant impact shaping more adaptive and intuitive user experiences. While machine learning algorithms will subsequently get smarter and can analyze user interactions in real time, they will make interfaces and content more dynamic. However, at this level of adaptability the user will be receiving experiences tailored to their unique preferences and needs, ultimately enhancing satisfaction and engagement.
In addition, integration of AI in UX research will encourage a shift toward more collaborative and inclusive design processes. Using AI tools to analyze different users perspectives and behaviours, companies can design for a broader user base. It also makes things more inclusive which not only increases the user satisfaction but also promotes innovation as it offers a wide range of ideas and feedback. The synergy between AI and UX research will thrive as we move forward, leading exciting and transformative changes in the user experience landscape.